Our principal engineer, Bart Kemper, P.E., presented at the IEEE 1st Workshop on Assured Autonomy, Artificial Intelligence, and Machine Learning (WAAM) on Oct. 31, 2022 in Charlotte, NC. It was held part of the larger 33rd IEEE International Symposium on Software Reliability Engineering (ISSRE). The paper’s topic, “AI and Stochastic Terrorism — Should it be done?“, is a topic developed with the event organizers to present an engineering ethics challenge regarding AI and machine learning (ML) that has not been significantly examine, such as AI in military applications. This topic is based on Bart’s past work in AI and critical infrastructure as well as his subsequent nomination to the National Institute for Standards and Technology’s (NIST) Subcommittee on Artificial Intelligence and Law Enforcement, coupled with his experience as a journalist, an anti-terrorism/counter-terrorism military professional, and a forensic engineer. This is an informal summary of the paper that does not violate IEEE’s copyright, along with additional background of the author.
Author’s Background
Before earning his engineering degree, Bart was a print journalist and worked his way through Louisiana State University’s engineering program working for the city papers and the wire services. He was an active member of the Society of Professional Journalists, the National Press Photographers Association, and the American Society of Media Photographers. He continued to freelance until 9/11, after which his Army Reserve duties moved to the forefront.
Bart originally joined the Army at the height of “Star Wars” era of the Cold War, then left to 82nd Airborne Division as a sergeant to earn his degree. He commissioned through LSU ROTC in 1992, choosing Reserve duty as an US Army Corps of Engineer officer. His father, Clarence Kemper, Jr. had commissioned as an Army engineer but became one of the first officers in the Military Intelligence branch when it was established in 1962 at Fort Holabird, MD. His life-long relationship with his father, coupled with growing up in different parts of the world in the 1960s, 70’s, and 80’s with active terrorism threats, gave Bart an early perspective on terrorism and its causes. This relation continued after Clarence’s retirement from the Army in 1988. Bart and his father co-founded Kemper Engineering Services in 2006 after Bart’s return from Iraq.
Another key perspective going to this paper is his own military career. While the “near peer” or “direct threat” has varied over time for Bart, the one continuous threat has been terrorism. While many focus on the larger, more existential threats, the reality of conflict is there is always irregular conflict, or “asymmetric warfare.” The variable is how much fighting there is between uninformed forces. While the Global War on Terror saw the use of Improvised Explosive Devices (IEDs) made by a small number of competent bomb makers such that it was worth the risk to have Explosive Ordnance Disposal (EOD) recover the weapons for forensic analysis, this was a theater-specific issue. With asymmetric conflicts like Vietnam, with a versatile adversary, or a traditional adversary with trained combat engineers, there is no value in risking EOD technicians unless the weapon cannot be blown in place for safety reasons. Engineers maintain the detailed expertise in explosives, including predicting its effects on structures such as explosive demolition operations as well as mines and roadside bombs, which are key methods are terrorist attacks. Retiring from the military in 2021, Bart’s background in anti-terrorism (preventing terrorism attacks) and counter-terrorism (actions against terrorists and their organization) spans the Cold War through the post-GWOT era. His final command was standing up and validating the 475th Engineer Detachment, Explosives Hazards Coordination Cell (EHCC) for the 412th Theater Engineer Command, in which his team was responsible for weapons intelligence gathering and analysis, targeting operations, blow-in-place (BIP) operations and training, and coordination between EOD, engineer, and supported forces. His military engineer career includes duty in Europe, Asia, Central and South America as well as combat tours in Iraq (Risk Mitigation and Reliability Lessons Learned From Iraq) and Afghanistan (Blast Modeling for Facility Security Management), the latter had him addressing a 61,000 pound IED, more than 10x the Oklahoma City Bombing and the largest IED recorded to date.
Finally, as a well-established engineering expert and Bart became a Board-Certified Forensic Engeer in 2017 through the National Academy of Forensic Engineer (NAFE). As his work in this sector grew, he became internationally certified as well as a member of the American Academy of Forensic Scientists (AAFS). Based on his scholarly work and past experience as a journalist, in 2020 he became the Editor In Chief of the peer-reviewed Journal of the NAFE, This was the final piece of the puzzle that led to the development of this paper. While his work in intelligence analysis and targeting gave him an intense understanding of the consequence of labels, his work in criminal and civil cases required Bart to understand and apply the Rules of Evidence and the various standards of care.
Paper Summary
This combinations of perspectives led to the paper exploring Stochastic Terrorism. The term was originally coined by Gordon Woo in 2002 in his paper, “Quantitative Terrorism Risk Assessment.” This addressed the manner that Al Queda broke from the traditional cell structure of terrorist groups and relied more on “inspired attacks” by leveraging social media and traditional news media. This concept uses Markovian chains and complexity theory and was further expanded in “Insuring Against Al-Queda” (Woo, 2003) and other works. Stochastic terrorism is still an actively used concept within risk assessment and anti-terrorism modeling and prediction circles. The computational principles involved does tend to limit its exposure outside of technical areas, but the principle behind it is clear: that stochastic terrorism is about evaluating the overall chance of a terrorist event, but it cannot be used ot predict a single event nor establish causational linkage between an attack and a source.
“The task of quantifying terrorism risk should not be confused with predicting the next terrorist attack. This important distinction between risk assessment and event prediction exists also with natural perils. No seismologist is capable of predicting the time, place and magnitude of the next major earthquake in California, but it is possible for a seismic risk analyst to evaluate the annual exceedance probability of loss to a California property portfolio.” “This distinction between risk assessment and event prediction matters to civic authorities and insurers. Being responsible primarily for public safety, civic authorities would ideally like to have reliable predictions to warn against imminent hazard events, or prioritize urgent defensive measures.. Insurers, on the other hand, seek to quantify risk not over a brief time window of a few days, but spread over a number of months. For this less ambitious purpose, quantitative risk assessment is achievable.” (Woo, 2002)
Traditional investigation and counter-intelligence means are far better suited to predict attacks in a specific place or to establish causation between an attack and a group or person. Terrorism inherently relies upon the societal impact of the attack more than the weapons effect of blast and shrapnel. Previous terrorist campaigns, such as the Irish Republican Army in the UK, the Red Brigade in Italy, and the Weatherman Underground in the US, often include other groups taking credit for a their actions or vice versa. The public credit for an attack is part of the cycle of inspiration that fuels support for a terrorist group but also increases the threat of government targeting for legal prosecution or military action. While there is certainly debate of the nuances of “what is a terrorist,” by statute and treaty it is an illegal combatant similar to a pirate. As such, labeling someone a “terrorist” is a targeting term similar to “murderer”, “rapist”, “child molester”, or cultural-specific terms such as “heretic” or “Nazi”, all of which signals it is acceptable to reject the person from society, possibly with direct violence similar to the “shoot on sight” order used with the Church of Latter Day Saints in the US or by citizens informing the police or military regarding targeted people.
Labels have meaning. Lynch mobs form and act based on a label being invoked. In terms of traditional journalism, it is important to differentiate allegations of an act from a conviction of the act. The factual basis and attribution of the basis is critical in traditional hard news reporting, which is about informing the public with minimal editorial commentary. In propaganda, the intent is to influence opinion to create outcomes. This includes political campaigns, commercial advertising, and official government media outlets. While limiting a campaign to “hard facts” is a technique, it is often not as effective in engaging emotions with editorial commentary and colorful prose.
A more colorful, non-technical use of “stochastic terrorism” developed later in the partisan publication Daily Kos, which describes itself is a progressive activist media outlet that lists “giving our readers the information they need to effectively engage in, and influence, the electoral process” as part it’s intent. “Our coverage is assiduously factual, ethical, and unapologetically progressive—there’s never a need to “both sides” the truth.” It is from this context a more political, non-technical use developed, in which a blogger was credited with inventing the term in 2011. While this new term shares the idea that “lone wolves” and other independent actors can be inspired to act, it goes further by labeling a pundit or political figure as directly responsible for the attack.
The idea of blaming a leader or a group for an attack is not new. In GWOT, arguably the most famous inspiration was Osama Bin Laden. A key factor in the assessment of an attack being “inspired” or not is whether the attacker states who their inspiration or source of information was as well as whether the speaker claims responsibility. It still needs corroboration, but in general its some form of admission. This non-technical use of stochastic terrorism uses an ispe dixit arguement , which loosely amounts to “because I said so”. In order to establish causation, there must be a substantial amount of evidence. In US jurisprudence there is a presumption of innocence in that the prosecution (criminal) or plaintiff (civil) must meet the burden of proof. This is where Artificial Intelligence and Machine Learning factor in.
Is is possible, if not probable, a jurisdiction would want to take action against those causing terrorism attacks. History is replete with authoritarian regimes using an ipse dixt arguement to convict dissidents of causing some form of unrest. This is more likely where there is no presumption of innocence. The question is whether there is a method to elevate “stochastic terrorism” to being more than an accusation of responsibility. Since this is rooted in social media, it requires an analysis of social media. ML is currently used to monitor social media for specific language cues, such as “hate language”. AI has similarly been used to analyze financial data for criminal activity. The technology is in place and the precedent of using computational tools is established in at least some jurisdictions.
In order to establish whether Pundit A caused Person X to act, it is necessary to establish two elements. The first element is whether Person X was exposed to the rhetoric of Pundit A. Pundit A cannot be logically the causation if Person X was not exposed. The other element is what other sources of inspiration had influenced Person X to act. Simple exposure cannot be sufficient as it would allow any recorded exposure, such as talk radio in a waiting room, to be attributed as causation despite the content being diametrically opposed to Person X’s political orientation. In a US jurisprudence model using broad strokes, Pundit A must be the predominant influence for Person X’s actions in a civil case. In a criminal case, there cannot be another attributable cause for Pundit A to be held directly responsible for Person X’s actions. Again, this is in broad strokes.
Social media is data. Theoretically, a machine learning model could examine years of social media records of Person X and other people of interest to build patterns of behaviour. AI would then process the data to assess what are the influences on Person X that are consistent with the events in question, to include returning a quantified assessment of these influences. At first glance, it seems like a reasonable use of existing technology. It would be lauded by those who wish to prevent future inspired attacks and would be likely to have a measure of popular support.
The first challenge is in the technical execution. Current AI models are prone to bias. Technology has not progressed to the point that bias can be reliably eliminated nor be readily assessed. The analysis would also be subject to bias based on the inputs. Like any other computational process, AI is subject to “Garbage In/Garbage Out” (GIGO). If the evidence is selected exclusively by one side or the other, there is likely bias in what data was selected for processing. There is also a separate issue of what would be the criteria for evaluating exposure and influence as well as how to weigh that against family upbringing, neural divergence, training & education, cultural norms, and other factors besides the data available in a social media analysis. Would a the accusation of “stochastic terrorism” only apply if an event occurred, or would there be criteria for an attempt similar to “conspiracy to commit murder” or even “attempted murder”?
The second challenge is systemic bias. The entity that will have access to a citizen’s internet exposure across various providers and platforms will be the government. The government has its own bias. History shows a government is more likely to see rhetoric enthusiastically supporting the government’s agenda as a positive thing whereas rhetoric enthusiastically criticizing the government will be seen as a negative. Governments have seen various calls for reforms and societal change, such as the labor movement and civil rights movement, in a negative light and made attempts to supress them. A government will be more likely to downgrade or excuse poor behavior, even violent behavior, in support of the government while being more likely to prosecute similar behavior that is against the government. This existing bias already creates a feedback loop that will strongly tend to reinforce the government’s interests. If a government funds the development of a ML/AI process to assess for stochastic terrorism, it is likely the system approved by the government will have a pro-government bias. In other words, the system is trained to be biased to support the government’s bias separately than the potential bias in data selection.
From a US perspective, these issues of bias may seem to be signficant barriers to developing computational tools to assess stochastic terrorism as a civil or criminal element of a charge. The principles of transparency, explainability, accountability, responsiblity, and assurance are critical in both the AI/ML field and in US jurisprudence. Expert witnesses using computational simulations must demonstrate their opinion is not a product of someone else’s programming. The liberal principles regarding free speech, freedom of association, and other rights within the legal system seem inconvenient with people “know” who is guilty and “deserves” punishment, but traditionally the US holds “it is better that 10 guilty people go free than one innocent person goes to jail.”
However, these cultural and legal barriers are not universal. The liberal concept of “free speech” may be seen as eroding in the US, but it is far less established in many other nations. Further, cultures that have greater value regarding conformity and domestic tranquillity will be more likely to introduce a similar bias into a computational model, similar to the “social score” in the People’s Republic of China. This is the crux of the ethical dillema for engineers and technicians in the AI and ML field: Just because a computational model can be developed, does it mean it should be? Would a “black box” be treated as “objective and unbiased” because it’s not a person, particularly if it aligns with popular confirmation bias?
There is irony that the accusation of being responsible for murder via stochastic terrorism may be, in and of itself, an act of stochastic terrorism. If a person is accused of murder or other crimes often enough, particularly in the context of “they are getting away with murder”, is it predictable someone would act outside the law in response to it? Arguably, this occurred in the 2017 Congressional baseball shooting where the shooter appeared to reacting to accusations against a political party.
It is noted the non-technical use of the term appears to be strongly aligned to the political leanings of the accuser when the political alignment is known. There is also irony that it probably an easier task to develop a ML/AI system for reviewing social media to confirm there is a link between a pundit and violent act, without examining for contrary causation, than to develop the statute for defining stochastic terrorism as an offense. The former is just programming, the latter would require people to consider “equality before the law.” The paper considers the growing use of AI and ML in the legal system and uses the issue of analyzing social media with respect to stochastic terrorism in the larger context of considering the potential impact of AI and ML in our society.
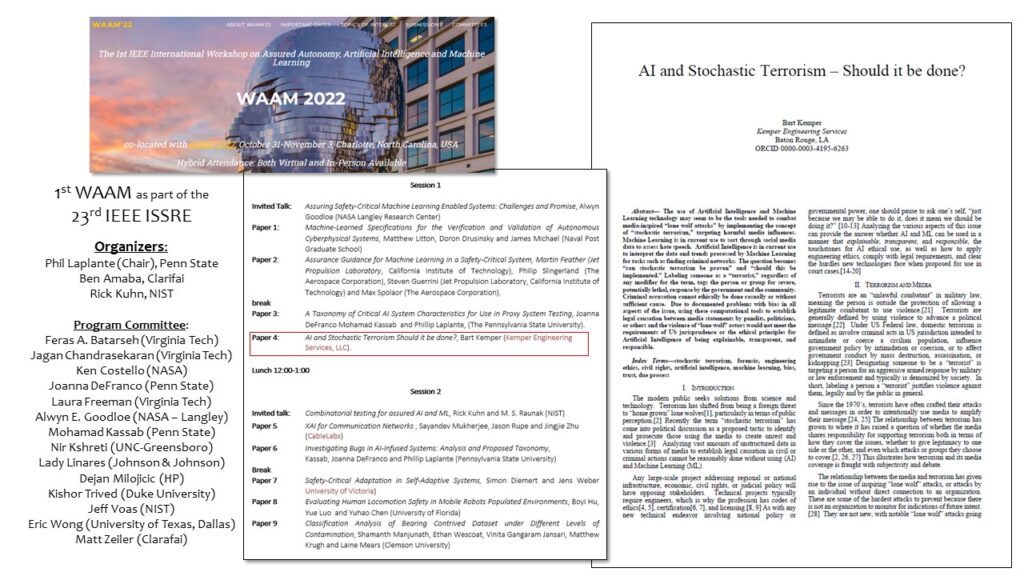
The papers presented at the IEEE 1st Workshop on Assured Autonomy, Artificial Intelligence, and Machine Learning (WAAM) as well as the organizing committee for this landmark event.
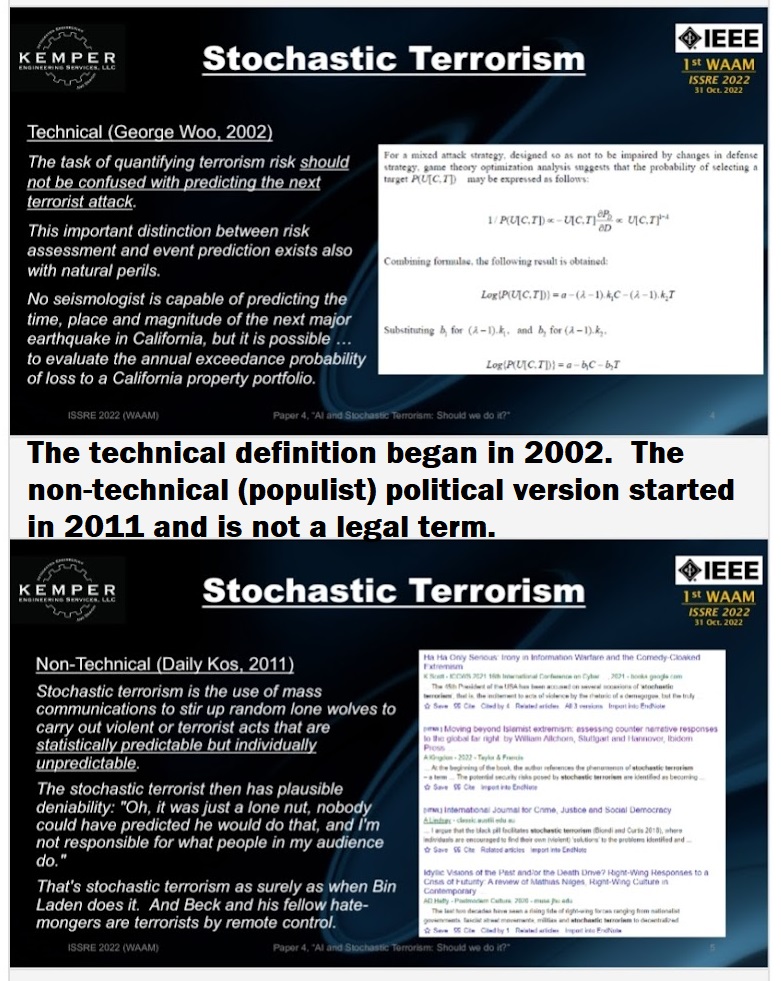
The technical and non-technical definitions for stochastic terrorism. While they agree on violence can be influenced by social media and other sources for rhetoric, the technical definition is about examining trends using specific numerical techniques. The non-technical usage assigns blame without a formal analysis or finding of fact.
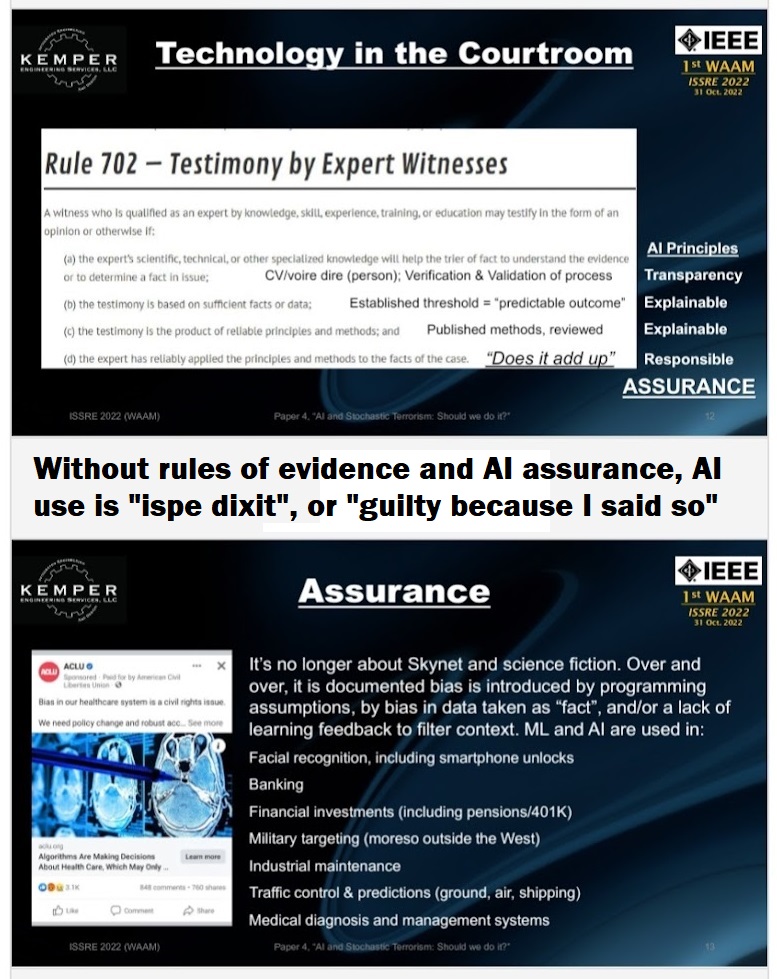
The federal rules of evidence have similar values as the principles for responsible Artificial Intelligence. A signficant challenge is that a human is understood to have bias, but a program is often perceived to be objective.
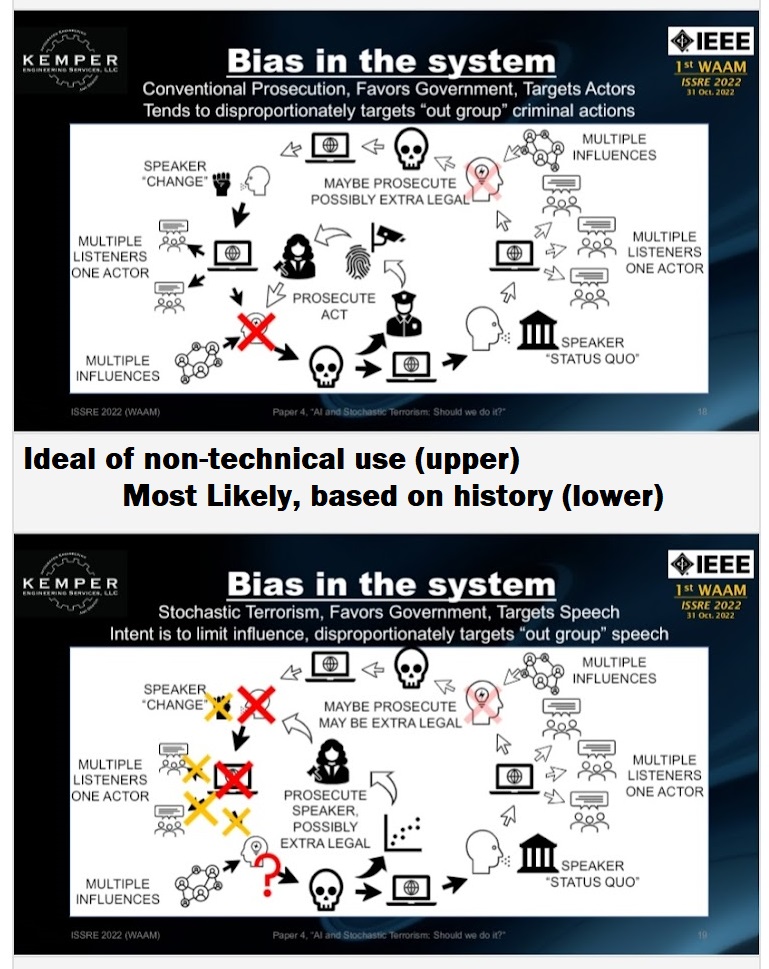
Theoretically, using AI and ML to analyze social media to assess for stochastic terrorism would be an objective process. The reality there is bias introduced in multiple ways, to include the typical bias a government has with respect to its own interests. Advocates for change and reform historically faced suppression. Engineers and technicians should carefully consider not just whether something can be developed, but should it be.
Recent Comments